Lemma 3.15. (Vitali type covering lemma. ) Let
be a collection of open balls in
, and let
. If
, there exist disjoint
such that 
Proof. The set
is open, then for
there exists a compact set
such that
.
Since
is compact, there exists finitely many balls
such that
. Let
be the collection of the balls. Choose
that has the largest radius. Let
be the collection of balls from
that is disjoint from
. Choose the ball
that has the largest radius. In general, suppose that
are choosen. Let
be the collection of balls in
that are disjoint from the previous
balls
. Choose
that has the largest radius. Since there are only
balls, the process will stop. We assume that
are the finally chosen balls. Given any ball
, we claim that
will intersect with some ball from
. Otherwise, one more ball will be chosen. A contradiction. Hence
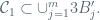
So
.
Definition. A measurable function
is called locally integrable with respect to the Lebesgue measure if
for every bounded measurable set
The space of such functions is denoted by 
Definition. If
, we define
.
Lemma 3.16. If
,
is jointly continuous in
.
Proof. Given
. Since
,
. By Corollary 3.6, given
, there exists
such that if
,
So given
, if
,
.
So
So

Hence as
,

Definition. If
, the Hardy-Littlewood maximal function
by

Remark. The function
is measurable for

is open for any
.
Theorem 3.17. There is a constant
such that for any
and
,
.
Proof. Let
. For
, there exists
such that
.
Let
. For any
, by Vitali type lemma 3.15, there exists disjoint balls
,

Note that the right hand side does not depend on
. Let
, we prove that

This proves Theorem 3.17.
We shall use the notion of limit superior for real valued functions of a real variable.

So
is equivalent to 
Theorem 3.18. If
, then
.
Proof. Since we are investigating continuity of a funtion at a point, it is a local property. So we may assume that
. Secondly, it is easy to see that the claim is true for a continuous function.
Now given
, there exists a continuous function
such that
Consider


Hence 
Given any
,

By the maximal theorem,

Let
, we see that
. We rationalize
, we see that

Definition. For any
, define the Lebesgue set
of
to be
.
Theorem. If
, then 
Proof. For any
,
. Then for
,

Let
. Then 
For any
, there exists
such that
,

So 
Let
,

Thus
. Since
, 
Definition. A Borel measure
is called “regular" if (i).
for every compact
. (ii).
, for any
. A signed measure or complex measure
is called
“regular” if
is regular.
Theorem 3.22. Let
be a regular signed or complex Borel measure on
, and
be its Lebesgue-Radon-Nikodym representation. Then for
,
.
Proof. We make two observations. (i). If
is regular, so are
. (ii). We may assume that
, because
.
We know that
are mutually singular. Let
be a Borel set such that
Let
. We will show that
for all
, which will complete the proof with the aid of the Lebesgue-Radon-Nikodym theorem.
By the regularity of
, for any
, there exists open set
such that
and
For any
, there exists
such that
. Let
and
, there exists
and disjoint balls
such that


Let
. We see that
. Since
,
. So 
Theorem 3.23. Let
be increasing, and let
.
(a). The set of points at which
is discontinuous is countable.
(b).
and
are differentiable
, and 
Proof. (a). Let
. It is clear that
is the set of points at which
is discontinuous. Since
is increasing, for
,
Also for
, the interval
contains a rational number. So
is at most countable.
(b). We observe that
is increasing and right continous.
except perhaps where
is discontinuous. Moreover
![G(x+h)-G(x) = \begin{cases} \mu_G((x,x+h]), \text{ if }h>0, \\ -\mu_G((x+h,x]), \text{ if }h<0\end{cases}.](https://s0.wp.com/latex.php?latex=G%28x%2Bh%29-G%28x%29+%3D+%5Cbegin%7Bcases%7D+%5Cmu_G%28%28x%2Cx%2Bh%5D%29%2C+%5Ctext%7B+if+%7Dh%3E0%2C+%5C%5C+-%5Cmu_G%28%28x%2Bh%2Cx%5D%29%2C+%5Ctext%7B+if+%7Dh%3C0%5Cend%7Bcases%7D.+&bg=ffffff&fg=4b5d67&s=0&c=20201002)
We know that
is regular. So by Theorem 3.22,
exists
.
Let
. We need to show that
exists and equals zero
Let
be an enumeration of points at which
, i.e.,
. Given any
,

Let
. Then
is a Borel measure that is finite on compact sets by
. Then
is a Lebesgue-Stieltjes measure.
We know that
. This goes to zero as
for
by Theorem 3.22. Indeed, let
. Then
since
is at most countable;
. So
. Thus
exists
, and
Definition. If
and
, we define

is called the total variation of $F$.
Remark. It is easy to observe that for $a<b$,
Thus
is an increasing function with values in
.
Definition. (1). If
,
is said to be of bounded variation on
. We denote the space of all such functions by
.
(2). The supremum in
is called the total variation of
on
. If it is finite, then we say that
.
Examples. (1). If
is bounded and increasing, then 
(2). If
and
, then 
(3). If
is differentiable on
and
is bounded, then
. Hint: using the mean value theorem.
(4). If
, then
for
, but
One can see it by taking
.
(5). If
for
and
, then
for
. One can see it by taking
.
Lemma 3.26. If
and is bounded, then
is increasing.
Proof. If
, then by
,
.
Theorem 3.27. (1).
iff
.
(2). If
, then
iff
is the difference of two bounded increasing functions. For the
direction, we take
.
(3). If
, then
exist for all
. This follows from (1) and (2).
(4). If
, the set of points at which
is discontinuous is countable. This follows from (1) and (2).
(5). If
and
, then
exist and are equal a.e. This follows from (1), (2) and Theorem 3.23.
Definition. NBV=\{F\in BV:\, F \text{ is right continuous and } F(-\infty) =0\}.
Remark. If
, then the function
defined by
is in NBV and
. Indeed,
; so
the difference of two increasing functions.
is again the difference of two increasing functions. So
.
is obvious.
Theorem 3.28. If
, then
. If
is also right continuous, so is
.
Proof. Given
and
, then by the definition of
, there exists
such that
So by
,
. This proves that
Since
is increasing,
for all
. So
.
For the second claim, we define
and assume that
. For any
, since $F$ is right continuous and
is defined, there exists
such that for
,

For any such
, there exists
such that
, and so

Similarly on the interval
, we apply the same reasoning to conclude that there exists
so that
.
So on
,

On the other hand, 
So that
. Since
is arbitrary, a contradiction. Therefore
.
Theorem 3.29. If
is a complex measure on
and
, then
. Conversely if
, there is a unique complex Borel measure
such that
; moreover
.
Proof. Suppose that
is a complex measure. By decomposing into real and complex parts, and then considering the positive and negative parts of measures,
. Since
is a complex measure,
are finite positive measures. Let
. Then
are right continuous, increasing functions. Also
and
. By Theorem 3.27 (1) and (2), 
Conversely, for
, we write
by Theorem 3.27 (1) and (2). Then by Theorem 3.27, each
is bounded and increasing. Then
. By Theorem 3.28,
is right continuous and so $T_F$ is right continuous. So each
is right continuous by Theorem 3.28. Also it is easy to see that each
by Theorem 3.28 again. So by Theorem 1.16,
for some finite Borel measure
. Let
.
The last step is to show that
. It is contained in Exercise 28 and 21 in Folland’s book.
Theorem 3.30. If
, then
. Morevoer
iff
, and
iff 
Proof. Since
, then $\mu_F$ in Theorem 3.29 is a complex Borel measure that is also regular. Then by the Radon-Nikodym theorem,
, where
, where
by Theorem 3.22. The rest conclusions follows easily from the Radon-Nikodym representation above.
Proposition 3.32. If
, then
is absolutely continuous iff
.
Proof.
If
, then by Theorem 3.5, we see that the claim holds.
We need to prove that for
, if
, then
. Since
is absolutely continuous, then for any
, there exists
such that for any finite disjoint intervals
, then if
,

Since
, there exists a sequence of open sets
such that
,
latex \mu_F$ is regular, we can find a sequence of open sets
such that
such that
. That is to say,
as
. Therefore
is a sequence of open sets that are decreasing and contains
, and moreover
. We abbreviate it by
.
Each
is an at most countable union of disjoint open intervals
. For any
,

So
Since
, we see that
Since
is arbitrary,
.
Corollary 3.33. If
, then the function
is in NBV and is absolutely continuous, and
Conversely if
is absolutely continuous, then
and
.
Proof. Suppose that
. Then
, right continuous and
by dominated convergence theorem. So
That
is absolutely continuous follows from Corollary 3.6. By Theorem 3.32, the deduced measure
and also
.
by Theorem 3.30. On the other hand,
gives rise to the same function
. By the uniqueness in Theorem 3.29, $fdm$ and $F’dm$ are the same complex Borel measures. Hence 
Conversely, if
and is absolutely continuous, then
by Theorem 3.32. Hence by Theorem 3.30,
Considering the real and imaginary parts of complex valued functions, and positive and negative parts of real-valued functions, we see that
because
is a complex Borel measure.
Lemma 2.34 If
is absolutely continuous on
, then
.
Proof. We know that
is absolutely continuous. Let
be given. There exists
such that for disjoint intervals
with
, then

Let $N$ be the smallest integer that is larger than
. We divide
into
consecutive segments with length that is at most
. For any points
, if necessary adding more endpoints of the previous consecutive segments , so that these points can be grouped into
subgroups. On each subgroup, if the points are denoted by
,
So the total sum is majorized by
This holds true for any partition of
. So
.
Theorem 3.35 (The fundamental theorem of calculus for Lebesgue integrals.) If
and
, the following are equivalent:
(a)
is absolutely continuous on $[a,b]$
(b)
for some
.
(c)
is differentiable
on
,
, and
.
Proof.
By subtracting
and extending to
trivially, we may assume that
by Lemma 3.34. So by Corollary 3.33,
follows.
is trival.
Extending
trivially to
, i.e.,
. Then we invoke Corollary 3.33.